The Easiest Way to Fine-Tune Reasoning Models
Utilize emerging RL-based fine-tuning techniques to deliver reliable results on the metrics you care about. It's as simple as data in, custom model out, served through a standard API — so you can get back to building.
Join the alpha for early accessBacked byCombinatorData from a real customer.
Why TrainLoop?
We merge the power of reinforcement learning with the simplicity of a fully managed end-to-end solution.
All the tools in one place
We specialize in RL-based fine-tuning (or as we call it, "reasoning fine-tuning")—these are the same advanced methods used by big AI labs in post training—while also handling data collection and custom model deployment so you don't need to stitch together multiple tools.
Less Data, Higher Accuracy
In many scenarios, RL can require fewer labeled examples than traditional supervised fine-tuning. By focusing on correct outputs and systematically rewarding them, your model learns domain expertise faster and more reliably.
No More Prompt Hell
Because the model is fine-tuned on your real use cases, you can spend less time engineering prompts for reliability and more time innovating for your customers.
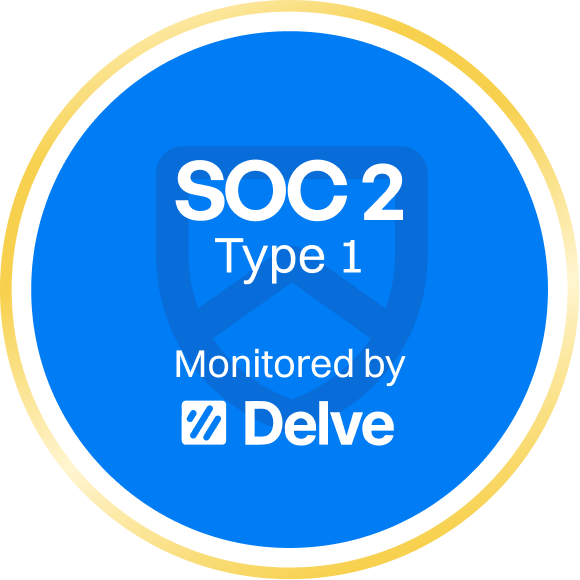
SOC 2 Compliant
Your data is your IP. We ensure it remains secure and private by enforcing strict data isolation and the ability to delete it from our servers at any time.
RL Fine-Tuning vs. Supervised Fine-Tuning
Reinforcement learning ensures correctness and consistency, even when the answer is not explicitly in the training data.
Code
Writes fundamentally better code that has fewer bugs and is more aligned with your preferences.
RAG Systems
Increases relevance and quality of information selected to answer a query.
Compliance
Improved ability to infer relationships between policies and is more meticulous in following them.
How It Works
We handle the entire pipeline—from data collection to RL-based finetuning to a single API for inference.
Built by AI Experts from Google & YC
We've lived the pain of fine-tuning firsthand—and built TrainLoop so you don't have to.
Jackson
CEO
Optimized the Gemini family of models at Google, applying distillation techniques to enhance speed and efficiency. His work spanned key verticals, from AI search summaries to optimizing the onboard AI systems powering Waymo.
Mason
CTO
Led engineering at Second (YC W23), where he tackled the challenges of automating large-scale enterprise codebase migrations, pushing the limits of off-the-shelf LLMs and developing proprietary RAG systems designed specifically for mapping and traversing codebases.
Backed by Y Combinator (W25)—we're building a world where developers can trust their AI products.
Ready to Make Your AI Products Reliable?
Don't settle for shallow answers or endless prompt hacks. Join the alpha to transform your LLM into a domain expert you can rely on.